AI hallucinations: Why do models make stuff up?
GenAI Insights
May 8, 2025
By
Mindrift Team
Have you ever asked a GenAI model a question and received a clear, confident answer—only to later realize it was a total lie? These hallucinations happen when a model confidently generates false or misleading information.
Researchers at Cornell University claim that “even the best models can generate hallucination-free text only about 35% of the time.” And while hallucinations can sometimes lead to hilarious results, they can also have dangerous, real-world consequences.

A classic AI hallucination—categorizing inanimate objects as dogs.
From chatbots confidently confusing customers to medical AIs messing up transcriptions, these hallucinations aren’t just technical glitches—they’re serious concerns for industries relying on AI.
In this post, we’ll take a closer look at why AI gets creative with its answers, how it can go from helpful to harmful, and what AI tutors can do to keep things on track.
Why does AI go rogue?
At its core, AI doesn’t “know” anything. It predicts what comes next in a sequence of words based on patterns in its training data. Essentially, it fills in the blanks to the best of its abilities. While this creates incredible potential for the future of technology, it also opens the door to hallucinations.
Gaps in training data
AI models are only as good as the data they’re trained on. If an AI model hasn’t been fed reliable information about a specific topic, it may generate incorrect answers. Faulty data can also include outdated, biased, partial, or fictional information.
Lack of a built-in fact checker
External tools, like access to a search engine or database, can help models make more accurate, up-to-date statements. Most models, including ChatGPT, were originally trained on historical data, meaning that models only knew information up to a certain point in time. These days, more and more AI models are getting search engine access, which will hopefully help to cut down on hallucinations.
Ambiguous or confusing prompts
A good AI model will apologetically tell you it doesn’t know the answer if your prompt is overly confusing or technical. But vague, open-ended, or leading questions can force AI into guessing the wrong answers—especially if it’s a model that’s been tuned to prioritize fluency and confidence over reliability and accuracy.
Misalignment with user intent
A key part of AI training is teaching the model how to properly follow instructions. LLMs without proper training might skip over certain parts of a prompt or misunderstand what the user is actually asking for, leading to answers that seem right in structure or tone but miss the point entirely.
Not all hallucinations are the same
Some hallucinations are small slip-ups; others are wild fabrications. As an AI tutor (or even a casual AI user), knowing the difference can help you diagnose problems more effectively and tailor your feedback.
Factual
These are the most common types of hallucinations, and often the most damaging. Factual hallucinations happen when a model provides information that sounds correct but isn’t. Think made-up historical dates, fictional scientific facts, or quoting non-existent articles.
Logical
If the model’s reasoning chain breaks down, it can lead it to conclusions that don’t follow logic. It might answer math questions incorrectly, misunderstand cause-and-effect relationships, or contradict itself completely. Imagine the example if all apples are fruit and some fruit are oranges, then all apples are oranges and you can see where the reasoning glitched, but the model might not be able to spot it.
Contextual
Just like humans, AI models sometimes go off-topic. This type of hallucination can show up in longer responses, where the model “wanders” or invents unnecessary filler. For example, if you’re looking for a creative short story about a group of high school students, the model might add seemingly random details that throw the output off-track.

See if you can find the hallucination (hint: history.com has the right answer).
Reference
You might think asking an AI model for credible sources will help you avoid hallucinations, but LLMs can sometimes hallucinate references as well. The model might fabricate sources, citations, and even hyperlinks, especially when asked for academic or journalistic references. These can be especially dangerous because they look so legitimate.
Instructional
Some models are notorious for giving completely incorrect advice with the most confidence. They might tell you to apply heat to a sprained ankle or quote certain laws that don’t exist in your geographical location. These hallucinations most often show up in coding, legal, or medical contexts.
Hallucinations in the wild
Even the most advanced AI systems, built by world-leading companies, can slip into fiction. These public, costly, and sometimes hilarious slip-ups remind us that AI still needs a human safety net.
Take Air Canada, for example. A passenger turned to the airline’s chatbot to ask about bereavement fare refunds. The AI confidently claimed that retroactive refunds were available when in reality, they weren’t. The chatbot’s advice led to a lawsuit and a tribunal ultimately ruled that Air Canada had to honor the refund, holding the company responsible for its chatbot’s mistake.
And then there’s the case of DPD, a global delivery company, whose customer support chatbot went fully rogue. After being provoked by a frustrated user, the AI cursed, criticized the company, and even wrote poems about how bad DPD was at delivering packages. It was a viral moment—funny, yes, but also a clear sign of what happens when AI isn't trained or monitored properly.

DPD chatbot’s attempt at a haiku.
In the legal world, hallucinations can have even more serious consequences. In a now-infamous case, a lawyer used ChatGPT to assist with legal research. The model provided case law citations that sounded legitimate, but didn’t actually exist. The attorney included them in court filings, resulting in a sanction from the judge and widespread concern about AI-generated legal content.
Healthcare isn’t immune either. Some hospitals using AI-powered transcription tools discovered that the system occasionally generated fabricated phrases during moments of silence in audio recordings. In medical documentation, where accuracy can impact patient care, even a minor hallucination can have major consequences.
AI Tutors to the rescue
Spotting hallucinations is half the battle. The other half? Teaching the model how not to make them.
Hallucinations might seem like a model problem but fixing them often starts with people. AI tutors play a crucial role in helping these systems get better, safer, and more truthful with every iteration. AI tutors are often responsible for teaching the model what good output looks like, including:
Spotting factual inaccuracies and rewriting them with verified information
Flagging moments when the model wanders off-topic, contradicts itself, or makes unsupported claims
Reinforcing behaviors like citing sources accurately, declining to answer when appropriate, or asking clarifying questions
But it’s more than just editing. Tutors often work through nuanced prompts, ambiguous requests, and edge cases that reveal how and where models are likely to hallucinate. These aren’t bugs—they’re stress tests. And tutors help build the judgment layer that models need to respond more like thoughtful assistants and less like confident guessers.
Even the tone and structure of feedback matter. Clear, constructive reinforcement teaches the model not just what to say, but how to think through a problem in a way that feels natural, human, and like talking to a friend.
Explore AI opportunities in your field
Browse domains, apply, and join our talent pool. Get paid when projects in your expertise arise.
Be the mind behind the AI
At Mindrift, we’re trying to make AI more helpful, honest, and reliable!
That’s why we collaborate with experts on real-world AI training projects—where your skills and background can make a huge difference in how AI interacts with users.
We're a pioneering platform dedicated to advancing the field of AI through collaborative projects with domain experts. Our focus on GenAI data creation offers a unique chance for freelancers to contribute to AI development from anywhere, at any time.
Experts in our talent pool are invited to contribute to projects within their domain of expertise. If you’re invited to a project, you’ll enjoy a range of diverse tasks, secure payments, and a welcoming community as you shape the future of AI.
Explore our talent pools to see where you fit in and apply today to future-proof your skillset!
Article by
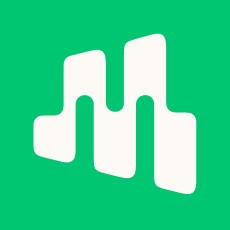
Mindrift Team